Analyse prédictive
3 choses à savoir
L’analyse prédictive permet de prévoir des événements futurs à partir de données historiques. Les données historiques sont généralement utilisées pour créer un modèle mathématique permettant de capturer les tendances importantes. Ce modèle prédictif est ensuite utilisé sur les données actives pour prévoir ce qui va se produire, ou encore pour suggérer des mesures à prendre afin d’optimiser les résultats.
Les analyses prédictives ont fait l’objet d’une grande attention au cours des dernières années en raison des progrès enregistrés dans les technologies qui le supportent, en particulier dans les domaines du Big Data et du Machine Learning.
Essor du Big Data
On entend souvent parler de l’analyse prédictive dans le contexte du big data. Les données d’ingénierie, par exemple, proviennent de capteurs, d’instruments et de systèmes connectés du monde entier. Dans une entreprise, les données système peuvent inclure des données transactionnelles, des résultats commerciaux, des réclamations clients et des informations marketing. Les entreprises s’appuient de plus en plus sur ces informations précieuses pour prendre des décisions.
Augmentation de la concurrence
Confrontées à une concurrence de plus en plus rude, les entreprises sont à la recherche de solutions pour commercialiser leurs produits et services sur des marchés saturés. Les modèles prédictifs reposant sur des données peuvent aider les entreprises à trouver de nouvelles méthodes pour résoudre ces problèmes de longue date.
Les fabricants d’équipements, par exemple, peuvent être confrontés à des difficultés pour innover au niveau du matériel. Les développeurs de produits peuvent ajouter des fonctionnalités prédictives aux solutions existantes afin d’augmenter la valeur ajoutée proposée au client. L’utilisation de l’analyse prédictive dans le cadre de la maintenance des équipements, ou maintenance prédictive, peut permettre d’anticiper les pannes des équipements, de prévoir les besoins en énergie et de réduire les coûts d’exploitation. Par exemple, les capteurs qui mesurent les vibrations dans les composants automobiles peuvent signaler un besoin de maintenance avant que le véhicule ne tombe en panne sur la route.
Les entreprises peuvent également utiliser l’analyse prédictive pour établir des prévisions plus précises, par exemple lorsqu’il s’agit de prévoir la demande en électricité au niveau du réseau de distribution électrique. Ces prévisions permettent d’optimiser la planification des ressources (planification de plusieurs centrales, par exemple).
Technologies de pointe pour le Big Data et le Machine Learning
Pour tirer de la valeur du Big Data, les entreprises appliquent des algorithmes à de grands ensembles de données à l’aide d’outils tels que Hadoop et Spark. Les sources de données peuvent être composées de bases de données transactionnelles, de fichiers de log d’équipements, d’images, de vidéos, d’audio, de données de capteurs ou d’autres types de données. C’est bien souvent l’association de données provenant de plusieurs sources qui rend possible l’innovation.
Avec toutes ces données, des outils sont nécessaires pour tirer des enseignements et des tendances. Des techniques de Machine Learning sont utilisées pour identifier des caractéristiques dans les données et concevoir des modèles qui prédisent les futurs résultats. De nombreux algorithmes de Machine Learning sont disponibles, notamment la régression linéaire et non linéaire, les réseaux de neurones, les machines à vecteurs de support, les arbres de décision, etc.
Exemples d’analyses prédictives
L’analyse prédictive aide les équipes dans des secteurs d’activité aussi divers que la finance, la santé, les produits pharmaceutiques, l’automobile, l’aérospatiale et la production industrielle.
- Automobile – Faire preuve d’innovation avec les véhicules autonomes
Les entreprises qui développent des technologies d’aide à la conduite et des nouveaux véhicules autonomes utilisent l’analyse prédictive pour analyser les données de capteurs des véhicules connectés et créer des algorithmes d’aide à la conduite. - Aérospatiale – Surveiller l’état des moteurs d’avions
Pour améliorer le temps de disponibilité des avions et réduire les coûts de maintenance, un fabricant de moteurs a créé une application d’analyse en temps réel afin de prévoir les performances des sous-systèmes dans les domaines suivants : huile, carburant, décollage, état mécanique et commandes. - Production d’énergie – Prévoir les prix et la demande en électricité
Les applications de prévision complexes utilisent des modèles qui permettent de surveiller la disponibilité des centrales, les tendances historiques, la saisonnalité et la météo. - Services financiers – Développer des modèles de risque de crédit
Les institutions financières utilisent des techniques de Machine Learning et des outils quantitatifs pour la prévision du risque de crédit. - Automatisation industrielle et machinerie – Prévoir les pannes de machines
Un producteur de films plastiques et de films minces économise 50 000 euros par mois en utilisant des applications de contrôle d’état et de maintenance prédictive qui permettent de réduire les temps d’arrêt et le gaspillage. - Appareils médicaux – Utiliser des algorithmes de reconnaissance de formes pour la détection de l’asthme et de la bronchopneumopathie chronique obstructive
Un appareil de gestion de l’asthme enregistre et analyse la respiration des patients et assure un retour instantané par l’intermédiaire d’une application pour smartphone qui aide les patients à gérer leur asthme et leur bronchopneumopathie chronique.
L’analyse prédictive est le processus qui consiste à utiliser l’analyse des données pour effectuer des prévisions en fonction des données. Ce processus utilise des données ainsi que des techniques d’analyse, de statistiques et de Machine Learning pour créer un modèle prédictif visant à prévoir les événements futurs.
Le terme « analyse prédictive » décrit l’application d’une technique statistique ou de Machine Learning visant à établir une prévision quantitative du futur. Souvent, des techniques de Machine Learning supervisé sont utilisées pour prévoir une valeur future (Pendant combien de temps cette machine peut-elle fonctionner avant qu’une maintenance soit nécessaire ?) ou pour estimer une probabilité (Quelle est la probabilité de défaut de remboursement de ce client ?).
Au départ, l’analyse prédictive est associée à un objectif commercial, qu’il s’agisse d’utiliser des données pour réduire le gaspillage, gagner du temps ou réduire les coûts. Le processus transforme des ensembles de données hétérogènes et souvent massifs en modèles capables de produire des résultats clairs et exploitables qui aident à atteindre cet objectif, qu’il s’agisse de réduire le gaspillage de matériau, de réduire l’inventaire en stock ou de fabriquer des produits conformes aux spécifications.
Processus d’analyse prédictive
Nous connaissons tous les modèles prédictifs utilisés pour les prévisions météorologiques. Une autre application sectorielle essentielle des modèles prédictifs concerne la prévision de la charge énergétique pour anticiper la demande en énergie. Dans ce cas de figure, les producteurs d’énergie, les opérateurs du réseau et les traders ont besoin de prévisions précises de la charge énergétique afin de pouvoir prendre des décisions concernant la gestion des charges dans le réseau électrique. Les volumes de données disponibles sont considérables, et grâce à l’analyse prédictive, les opérateurs du réseau peuvent les transformer en informations exploitables.
Figure 1. Processus d’analyse prédictive.
Processus détaillé de la prévision des charges énergétiques
Le prcoessus d’une application d’analyse prédictive suit généralement ces étapes principales :
- Importer des données à partir de sources variées, par exemple des archives Web, des bases de données et des feuilles de calcul.
Parmi les sources de données disponibles, citons les données de charge énergétique répertoriées dans un fichier CSV ainsi que les données météorologiques nationales indiquant la température et le point de rosée. - Nettoyer les données en supprimant les valeurs aberrantes et en combinant les sources de données.
Identifiez les pics de données, les données manquantes ou les points anormaux à supprimer des données. Ensuite, rassemblez les différentes sources de données (dans le cas qui nous intéresse, en créant un tableau unique incluant la charge énergétique, la température et le point de rosée. - Développer un modèle prédictif précis reposant sur les données agrégées en utilisant des statistiques, des outils d’ajustement de courbes ou le Machine Learning.
La prévision énergétique est un processus complexe avec de nombreuses variables, c’est pourquoi il peut être utile d’utiliser des réseaux de neurones pour créer et entraîner un modèle prédictif. Faites des expérimentations dans votre ensemble de données d’entraînement pour tester différentes approches. Une fois l’entraînement terminé, vous pouvez essayer le modèle avec de nouvelles données afin de savoir s’il fonctionne correctement. - Intégrer le modèle dans le système de prévision de charge d’un environnement de production.
Une fois que vous avez trouvé le modèle permettant de prévoir la charge de façon précise, vous pouvez le transférer dans votre système de production afin de rendre les analyses disponibles pour les programmes logiciels ou les appareils (y compris les applications Web, les serveurs ou les appareils mobiles).

Figure 2. Application d’analyse prédictive pour la prévision de la charge énergétique dans l’État de New York.
Élaboration de modèles prédictifs
Vos données agrégées racontent une histoire complexe. Pour extraire les informations qu’elles contiennent, vous devez disposer d’un modèle prédictif précis.
La modélisation prédictive utilise des méthodes mathématiques et de calcul pour prévoir un événement ou un résultat. Ce processus permet de prévoir un résultat à un stade donné ou en se basant sur une durée en fonction des modifications apportées aux entrées du modèle. À l’aide d’un processus itératif, vous développez le modèle en utilisant un jeu de données d’entraînement, puis vous le testez et vous le validez afin de déterminer sa précision pour effectuer des prévisions. Vous pouvez essayer différentes approches de Machine Learning pour identifier le modèle le plus efficace.
Il peut s’agir par exemple de modèles de régression de séries temporelles visant à prévoir le volume du trafic aérien ou le rendement énergétique en fonction d’un modèle de régression linéaire de la vitesse du moteur par rapport à la charge.
Comparaison de l’analyse prédictive et de l’analyse prescriptive
Les entreprises ayant réussi la mise en œuvre de l’analyse prédictive considèrent le passage à l’analyse prescriptive comme l’étape suivante. L’analyse prédictive crée une estimation de ce qui va se produire ; l’analyse prescriptive vous indique comment réagir de la meilleure façon en fonction de la prévision.
L’analyse prescriptive est une branche de l’analyse de données qui utilise des modèles prédictifs pour suggérer des mesures à prendre afin d’optimiser les résultats. L’analyse prescriptive s’appuie sur des techniques basées sur l’optimisation et les règles pour la prise de décision. La prévision de la charge sur le réseau électrique au cours des 24 heures suivantes est un exemple d’analyse prédictive, tandis que le fait de décider le mode d’exploitation des centrales en s’appuyant sur cette prévision est une application d’analyse prescriptive.
Exemples intéressants d’analyse prédictive avec MATLAB
Les entreprises trouvent des solutions innovantes pour appliquer l’analyse prédictive, en utilisant MATLAB® pour créer de nouveaux produits et services, ainsi que pour identifier de nouvelles manières de résoudre des problèmes auxquels elles sont confrontées depuis longtemps.
Les exemples suivant illustrent l’analyse prédictive en action :
Baker Hughes développe un logiciel de maintenance prédictive pour les équipements d’extraction de gaz et de pétrole à l’aide de l’analyse de données et du Machine Learning
Les camions Baker Hughes sont équipés de pompes à déplacement direct qui injectent un mélange d’eau et de sable en profondeur à l’intérieur des puits forés. Chacune de ces pompes coûte environ 100 000 dollars sur les 1,5 million de dollars que coûte un camion au total. Baker Hughes avait donc besoin de pouvoir déterminer à quel moment une pompe allait tomber en panne. L’entreprise a traité et analysé jusqu’à un téraoctet de données recueillies à 50 000 échantillons par seconde à partir de capteurs installés sur 10 camions opérant sur le terrain, et elle a entraîné un réseau de neurones afin d’utiliser les données de capteurs pour prévoir les pannes des pompes. Le logiciel devrait permettre de réduire les coûts de maintenance de 30 à 40 %, ce qui représente plus de 10 millions de dollars.
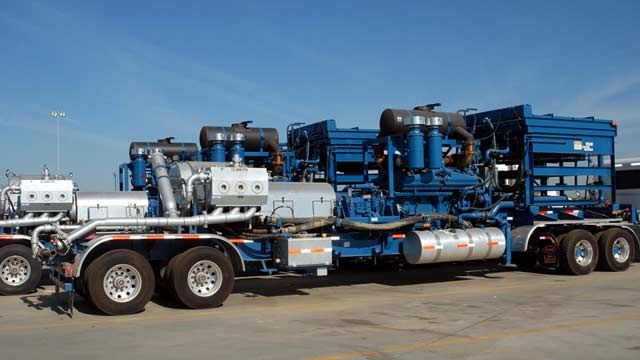
BuildingIQ développe des algorithmes proactifs pour l’optimisation de la consommation des équipements HVAC des grands bâtiments
Les systèmes HVAC (chauffage, ventilation et climatisation) des grands bâtiments sont souvent inefficaces, car ils ne prennent pas en compte les changements météorologiques, les coûts d’énergie variables ou les propriétés thermiques du bâtiment. La plate-forme logicielle basée sur le Cloud de BuildingIQ utilise des algorithmes avancés pour traiter en continu plusieurs giga-octets d’informations provenant d’instruments de mesure de puissance, de thermomètres et de capteurs de pression pour systèmes HVAC. Le Machine Learning est utilisé pour segmenter les données afin de déterminer les contributions relatives du gaz, de l’énergie électrique, de l’énergie thermique et de l’énergie solaire aux processus de chauffage et de refroidissement. L’optimisation sert à déterminer la meilleure planification pour le chauffage et le refroidissement de chaque bâtiment tout au long de la journée. La plate-forme de BuildingIQ réduit la consommation d’énergie des équipements HVAC des grands locaux commerciaux de 10 à 25 % en situation de fonctionnement normal.
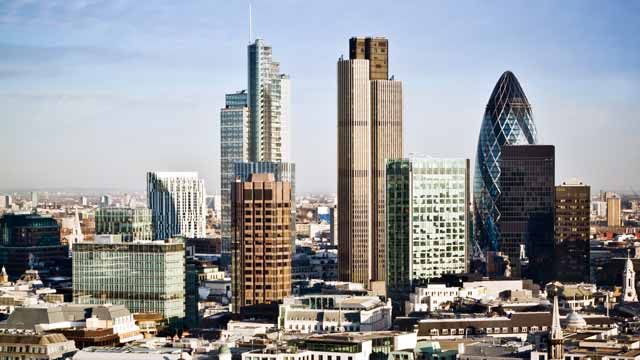
Développement d’algorithmes de détection visant à réduire le nombre de fausses alarmes dans les services de soins intensifs
Les fausses alarmes dues aux électrocardiographes et autres appareils de surveillance des patients représentent un sérieux problème dans les services de soins intensifs. Le bruit émis par ces fausses alarmes perturbe le sommeil des patients, et lorsqu’elles sont trop fréquentes, elles réduisent la capacité du personnel médical à réagir en cas de véritable alerte. Les participants au concours PhysioNet/Computing in Cardiology Challenge ont été chargés de développer des algorithmes capables de faire la distinction entre les vraies et fausses alarmes dans les signaux enregistrés par les appareils de surveillance des services de soins intensifs. Les chercheurs de l’Académie tchèque des sciences ont remporté la première place dans la catégorie « temps réel » du concours grâce à des algorithmes MATLAB capables de détecter les complexes QRS, de faire la distinction entre les battements cardiaque normaux et ventriculaires, ainsi que d’éliminer les « faux » complexes QRS dus aux stimuli des cardiostimulateurs. Les algorithmes ont permis d’obtenir un taux de vrais positifs et un taux de vrais négatifs de 92 % et 88 %, respectivement.
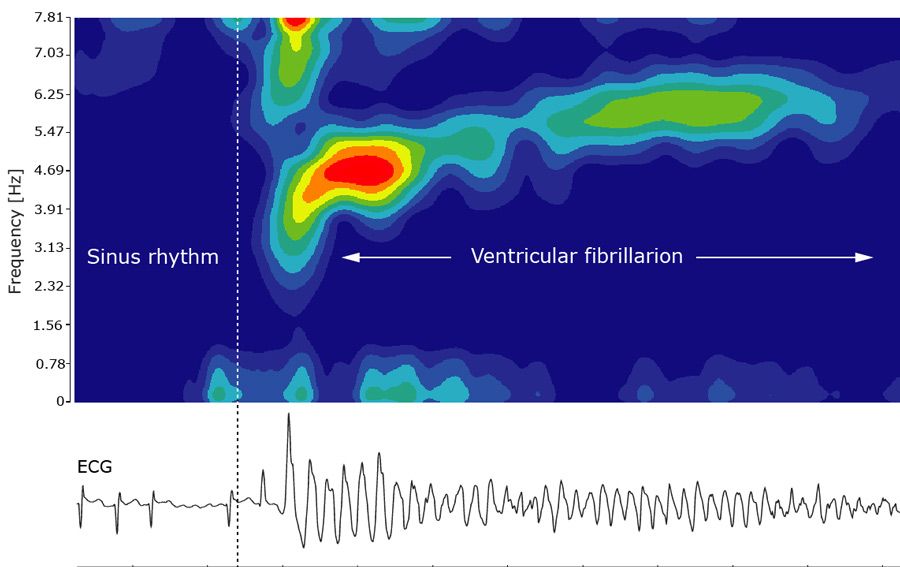
Pour tirer parti de la valeur des données commerciales et d’ingénierie afin de prendre des décisions éclairées, les équipes qui développent des applications d’analyse prédictive font de plus en plus appel à MATLAB.
Les outils et fonctions MATLAB permettent d’effectuer des analyses prédictives à l’aide de données d’ingénierie, scientifiques et de terrain, mais également de données commerciales et transactionnelles. MATLAB vous permet de déployer les applications d’analyse prédictive vers des systèmes de production à grande échelle et des systèmes embarqués.
Figure 3. MATLAB pour l’analyse prédictive.
Pourquoi utiliser MATLAB pour l’analyse prédictive ?
- Les analyses MATLAB utilisent à la fois des données commerciales et techniques.
MATLAB supporte nativement les formats de données issues de capteurs, les images, les vidéos, la télémétrie, les données binaires et autres formats temps réel. Explorez ces données au moyen des tableaux tall MATLAB pour Hadoop et Spark, ainsi qu’en connectant les interfaces aux bases de données ODBC/JDBC. - MATLAB laisse les ingénieurs faire eux-mêmes de la science des données.
Donnez à vos spécialistes du domaine l’opportunité de faire de la science des données, avec des outils puissants pour les aider à utiliser le Machine Learning, le Deep Learning, les statistiques, de l’optimisation, l’analyse des signaux et le traitement des images. - Les analyses MATLAB sont exécutées dans des systèmes embarqués.
Développez des analyses à exécuter sur des plates-formes embarquées, en créant du code C et C++ portable à partir de code MATLAB. - Les analyses MATLAB peuvent être déployées vers les systèmes informatiques d’entreprise.
MATLAB s’intègre dans les systèmes d’entreprise, les clusters et les Clouds, à l’aide d’un runtime déployable sans redevance.
Vos données + MATLAB = mise en œuvre réussie de l’analyse prédictive
Dans cet aperçu simplifié, les données techniques proviennent de capteurs, instruments et systèmes connectés du monde entier. Les données sont collectées et stockées dans un système de fichiers, que ce soit en interne ou dans le Cloud.
Figure 4. Architecture d’une analyse axée sur les données techniques.
« Peu importe le secteur d’activité de nos clients et les données qu’ils nous demandent d’analyser (texte, audio, images ou vidéo), le code MATLAB nous permet de fournir des résultats clairs, plus rapidement. »
Ces données sont associées à d’autres données provenant de systèmes métiers traditionnels, par exemple les données sur les coûts, les résultats commerciaux, les réclamations clients et les informations marketing.
Les analyses sont ensuite développées par un ingénieur ou un spécialiste du domaine à l’aide de MATLAB. Un prétraitement est presque toujours nécessaire pour gérer les données manquantes, les valeurs aberrantes ou autres problèmes imprévus de qualité des données. Ensuite, des méthodes d’analyse comme les statistiques et le Machine Learning sont utilisées pour produire une « analyse » (un modèle prédictif de votre système).
Pour être utile, ce modèle prédictif est ensuite déployé soit dans un environnement informatique de production alimentant un système informatique ou transactionnel en temps réel (par exemple un site de commerce électronique), soit vers un appareil embarqué (capteur, contrôleur ou système intelligent utilisé dans le monde réel, par exemple véhicule autonome).
L’application de MATLAB et Simulink® dans le cadre de cette architecture constitue l’approche idéale, car ces outils simplifient le déploiement vers les systèmes embarqués grâce au Model-Based Design, ou vers les systèmes informatiques grâce aux produits de déploiement d’applications.
Figure 5. Déploiement de modèles prédictifs vers des systèmes embarqués et des systèmes IT.
« MATLAB nous a permis d’accélérer notre R&D et notre déploiement grâce à la robustesse de ses algorithmes numériques, à ses outils exhaustifs de visualisation et d’analyse, à ses routines d’optimisation fiables, au support de la programmation orientée objet et à la possibilité d’exécuter dans le Cloud avec nos applications Java de production. »